Remember when Salvador Dali and Pablo Picasso decided to have a paint-off on the Starship Enterprise’s holodeck? No? Well, neither do we. But if you asked ChatGPT, there’s a good chance it would draft a rather vivid account of the encounter and claim it’s exactly how it happened. If you’ve never heard about AI hallucination, here’s everything you need to know.
💡 Before you start… New to AI? Make sure to check other articles in our AI series where we cover cool things like autonomous task management and the use of AI in note-taking.
🤯 Defining AI Hallucinations
Pythia was the high priestess of the Temple of Apollo at Delphi. The ancient Greeks believed the revered oracle could tap into the wisdom of the gods to provide advice and guidance. Fast forward almost three thousands years, AI is taking over that glorified position.
And the results?
Much more reliable that a self-professed mystic but still peppered with the occasional glitchy mishap that leaves you scratching your head. But let’s get serious for a moment.
In a nutshell, AI hallucinations refer to a situation where artificial intelligence (AI) generates an output that isn’t accurate or even present in its original training data.
💡 AI Trivia: Some believe that the term “hallucinations” is not accurate in the context of AI systems. Unlike human hallucinations, neural networks don’t perceive or misinterpret reality in a conscious sense. Instead, they blunder (aren’t we all?) due to anomalies in data processing.
Depending on the AI tool you use, hallucinations may range from simple factual inconsistencies generated by AI chatbots to bizarre, dream-like visions conjured up by text-to-image generators.
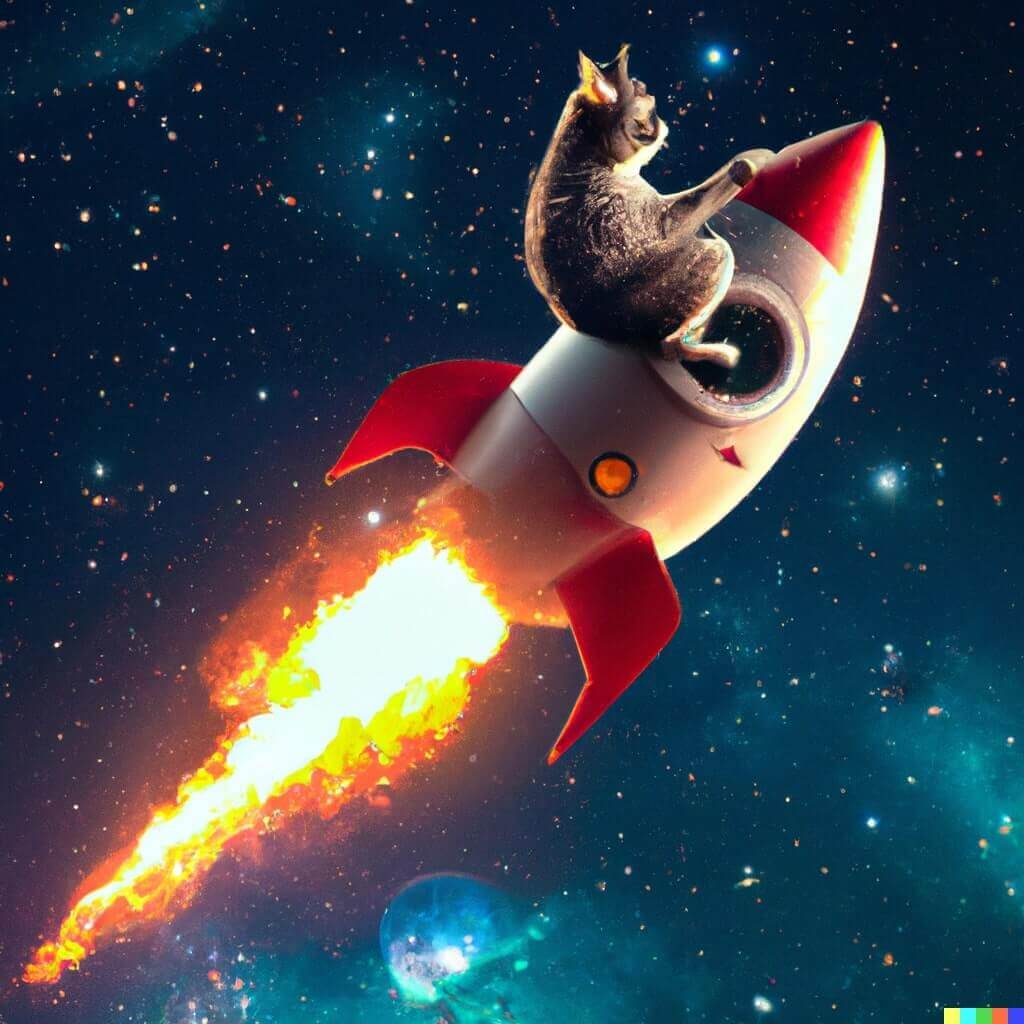
AI hallucinations happen to all AI models, no matter how advanced they are. The good news is there are ways to prevent or minimize them. But we’ll get to that in a moment.
First, let’s dig in and see what makes AI models tick.
🤖 Understanding AI and Machine Learning
Large language models (LLM) like GPT-3 or GPT-4 generate human-like text by predicting the next word in a sequence. Image recognition models classify images and identify objects by analyzing patterns in pixel data such as edges, textures, and colors. Text-to-image generators like DALL-E 2 or Midjourney transform a random noise pattern into visual output.
All those models use machine learning algorithms to parse data, learn from it, and make predictions or decisions without being explicitly programmed to perform the task.
Training artificial intelligence (read: feeding it a ton of data) can happen in several ways:
- 🟡 Supervised Learning: The algorithm learns from labeled training data. For example, it can learn how to differentiate a dog from a cat by looking at labeled photos of animals.
- 🟢 Unsupervised Learning: During unsupervised learning, the algorithm tries to find patterns in previously unseen data. It’s like exploring a new city without a guide.
- 🔴 Reinforcement Learning: The algorithm learns optimal actions based on positive or negative feedback, not unlike a pet that’s rewarded with treats for good behavior.
This process is called deep learning, a subset of machine learning that uses artificial neural networks to enable AI to learn, understand, and predict complex patterns. While the performance of a deep learning model depends on multiple factors, access to data is key.
And this takes us to our next point. 👇
⚡️ Causes of AI Hallucinations
AI models don’t have common sense or self-awareness (yet). And despite what many would want you to believe, they’re not really creative, at least not in the same way humans are.
The output you get from a tool like ChatGPT is solely based on patterns learned from training data. An AI model has no way of knowing whether the training data is correct or not. It can’t reason, analyze, and evaluate information, which means data is also the weakest link.
A simple illustration — an AI developed to classify musical genres, trained only on classical music, might misclassify a jazz piece. In a more serious case, a facial recognition AI trained exclusively on faces of a certain ethnicity may fail to recognize faces from other ethnic groups.
Of course, data is only part of the problem.
Communicating with A-powered tools involves what’s called prompt engineering. In a nutshell, prompt engineering is the process of writing instructions or “prompts” to guide AI systems.
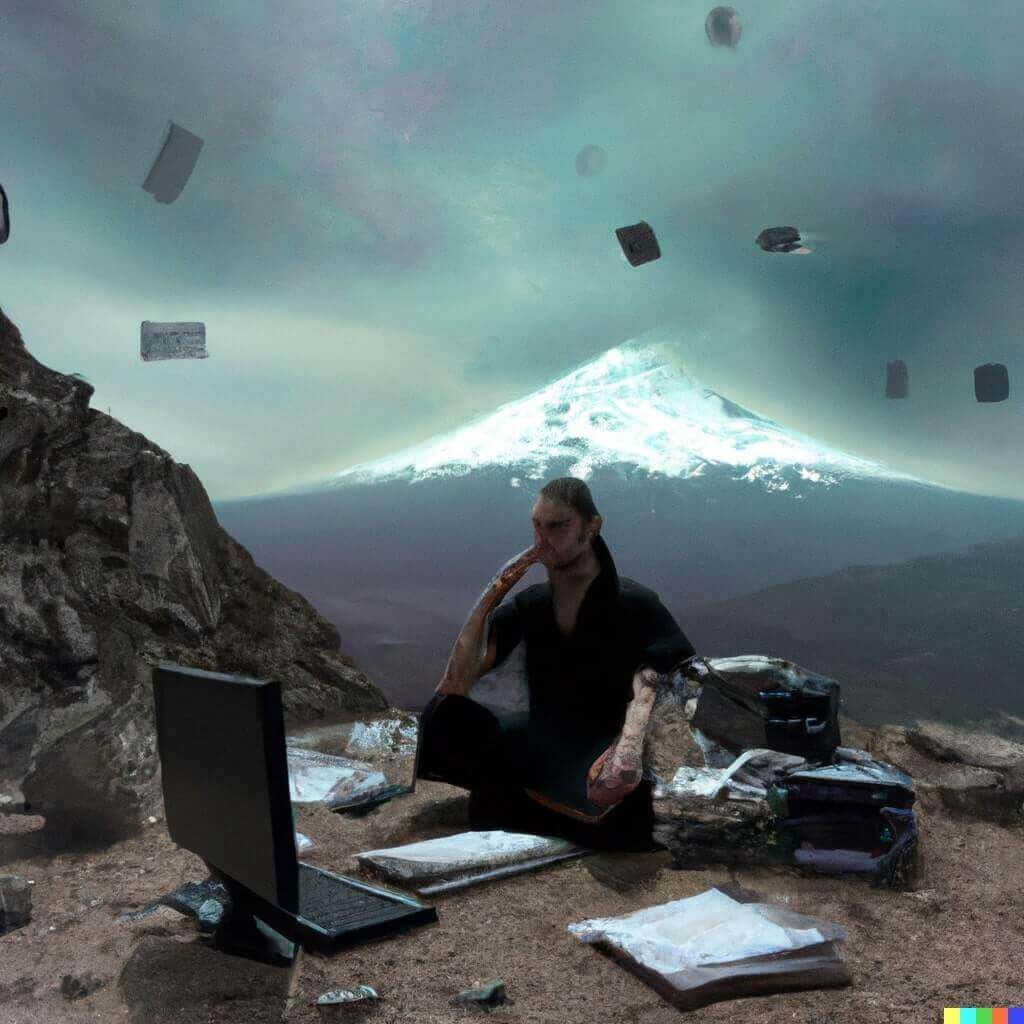
AI models are extremely capable of untangling the nuances of language. But they can’t magically interpret vague and ambiguous prompts with perfect precision. Prompts that lack precision, offer incomplete context, or imply facts can throw off AI output quite easily.
Sometimes, AI hallucinations are caused by the inherent design of an AI system.
An language model that’s closely tailored to its training data may start to memorize patterns, noise, or outliers specific to that data, a phenomenon known as “overfitting.” The model may perform well within the scope of its training data but will struggle in more general, creative tasks.
🤹 Examples of AI Hallucinations
Blending Fiction with Reality
AI chatbots like ChatGPT are superb conversation partners. They can come up with good ideas and provide a fresh perspective, unhindered by conventional thinking. Well, most of the time.
Since the knowledge of an AI model is limited to its training dataset, it may start improvising when it encounters tasks outside its “comfort zone.” The result? Fabricated data, made-up stories, and bizarre narratives where facts mingle with fiction, all pitched as absolute truth.
Claiming Ownership
Earlier this year, several online communities exploded with reports of teachers and university professors striking down students’ written works as generated by AI.
And the scrutiny and suspicion makes a lot of sense. After all, generative models are the low-hanging fruit for students who don’t feel like putting in the hard work.
The problem is that in many cases, professors attempted to blow the lid off the alleged AI cheating by feeding students’ papers back to ChatGPT and asking who wrote it (no doubt with a victorious grin). The AI would simply hallucinate and claim ownership like it’s no big deal.
Daydreaming
In 2015, Google engineer Alexander Mordvintsev developed a computer vision program called DeepDream that could recognize and enhance patterns in images. DeepDream used a technology called convolutional neural network (CNN) to create bizarre, dream-like visuals.
While AI image generation has made significant progress thanks to a new breed of diffusion models, AI hallucinations are still equally entertaining and unsettling at the same time.
Remember the 1937 musical drama Heidi starring Shirley Temple? A Twitter user by the name of @karpi leveraged AI to generate a trailer for the movie and the result was… well, unsettling. Think puffed-up cows, angry mobs, and an entire cast of anthropomorphic creatures.
Or just see it for yourself!
Reckless Driving
In the 1960s, Larry Roberts (known as the “father of computer vision”) penned a Ph.D. thesis titled “Machine Perception of Three-Dimensional Solids.” His work kicked off the development of image recognition technology, which is now used extensively in the automotive industry.
A decade later, The Tsukuba Mechanical Engineering Lab in Japan created the first self-driving car. The vehicle moved along a supporting rail and reached an unimpressive 19 mph.
Fast forward to 2023, self-driving cars are still a song of the future. Part of the reason for this slow adoption may be the fact that the National Highway Traffic Safety Administration reported 400 car crashes involving fully and semi-autonomous vehicles in the last 11 months.
(most accidents involved human error, which is comforting)
🤔 Impact and Implications of AI Hallucinations
Ok, AI hallucinations may seem surreal, fascinating, or terrifying. But for the most part, they’re relatively harmless (unless your car suddenly develops a personality at 70 miles per hour).
But the fact that AI can hallucinate poses a question — can we trust artificial intelligence?
Until last November, AI systems were working mostly behind the scenes, curating social media feeds, recommending products, filtering SPAM, or stubbornly recommending documentaries on competitive knitting after watching a single YouTube tutorial.
(and we thought we’ve seen it all)
As artificial intelligence is gaining more traction, an occasional blunder on a larger scale may erode what little trust and admiration we’ve managed to muster.
A 2023 study run by the University of Queensland and KPMG found that three in five people, or roughly 61%, are on the fence or straight-up not ready to trust AI. While 85% believe AI can be a good thing in the long run, we’re still wary of the risk vs. benefit ratio.
One of the major problems with AI models, especially conversational AI tools like ChatGPT, is that they tend to present “facts” with utmost confidence and not a shred of humility.
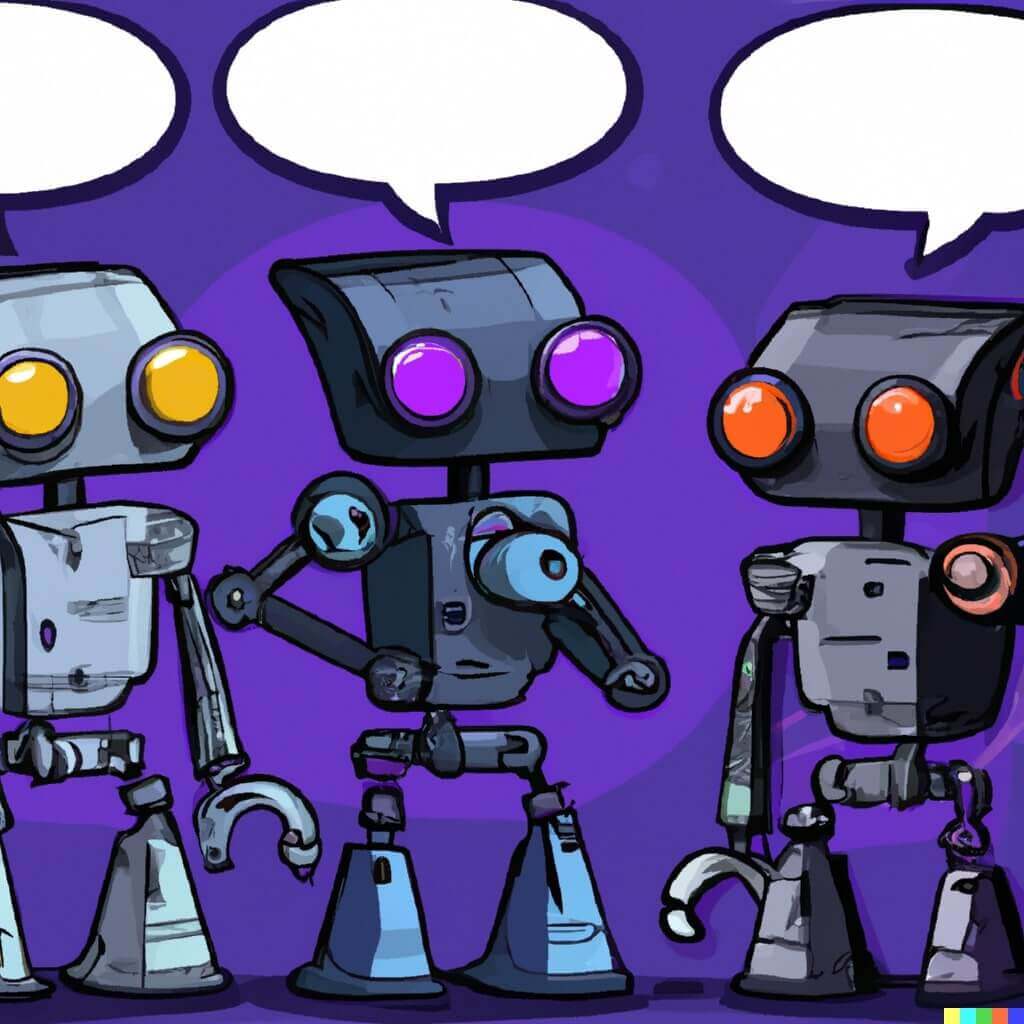
Granted, the quality of the information you can find online has been degrading for a long time. Even a diligent Google search is likely to give you a ton of irrelevant results, many riddled with low-quality content sprinkled with plagiarized or outright deceptive material.
But in the case of online resources, there are still ways to fact-check information. Ask AI for a source and it will simply invent it, going as far as hallucinating URLs of non-existent pages.
The good news is that the rate of hallucination for newer models has decreased significantly. According to OpenAI, GPT-4 is 30% less likely to hallucinate than its predecessor.
Of course, there are other problems — biased data, low diversity, lack of transparency, security and privacy concerns, just to name a few. All that is topped off with ethical dilemmas, legal conundrums, and other aspects of AI we, as a society, haven’t figured out yet.
Ok, so what can we do about it?
🛠️ Ways to Prevent AI Hallucinations
Good Prompt Engineering
While we may have little agency over the way an AI model is trained (unless you train it yourself), you can still steer it in the right direction with well-written prompts.
Here are a few tips that will help you get started:
- 🎯 Be specific: Avoid ambiguous language. The more specific your prompts, the less room there is for AI to hallucinate. Try not to overload your prompts with instructions. If possible, ask the AI to break the output into smaller steps.
- 💭 Provide context: The context of a prompt can include the expected output format, background information, relevant constraints, or specific examples.
- 🔗 Request sources: Ask the AI model to provide sources for each piece of information included in the output. Extracting accurate sources from an AI model may be tricky, but it will help you cross-check if the information is valid, reliable, and up-to-date.
- 🚦 Give feedback: Provide feedback when the AI hallucinates. Use follow-ups to point out any problems and use clarifying prompts to help AI rehash the output.
Need more tips? Check our guide to AI prompt engineering next.
Turn the Temperature Down
When an AI model generates predictions, it generates a probability distribution that represents the likelihood of different potential outcomes, such as words in a sentence. “Temperature” is a parameter that adjusts the shape of this distribution, making the model more (or less) creative.
The lower the temperature, the more likely the model is to return a “safe” (read: more accurate) output. The higher you set it, the higher the chances that it will hallucinate.
While ChatGPT has a predefined temperature setting that can’t be changed by the user, you control this parameter using OpenAI’s GPT-3/4 APIs or one of many open-source models.
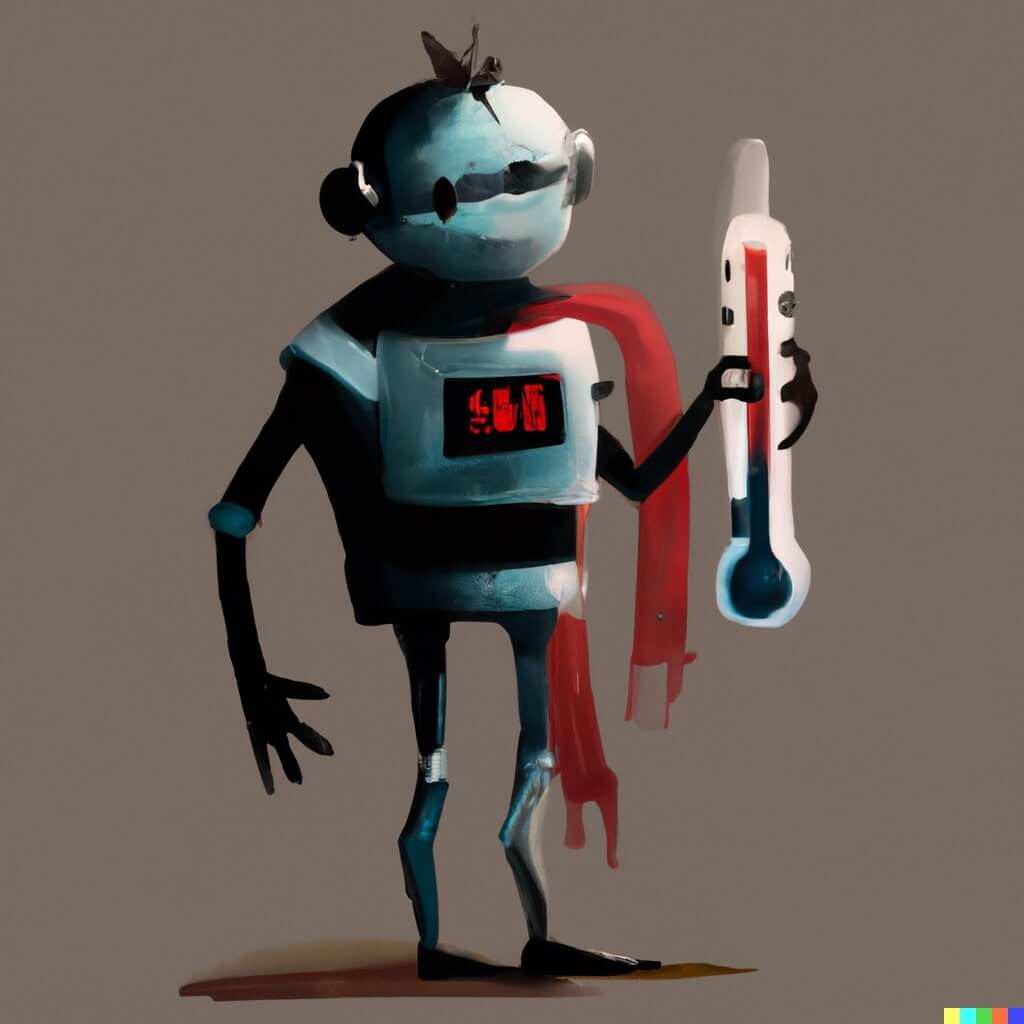
Provide Examples
Ok, this one is fairly simple to achieve if you already have some content on your hands.
For example, let’s say you’re writing an article in a specific style, language, or format. You can paste in the parts of the document you’ve written so far and ask AI to follow it.
The results won’t be a 10/10 match all the time, but they will be much better than what a generic prompt without a context or point of reference will give you.
You can do this with structured data too. If you want ChatGPT to generate and fill in a table, define the column labels you want to use and specify the number of rows / columns. Or, if you’re working with code snippets, simply provide a sample so AI can jump right in.
👋 Parting Words
AI tools, and conversational AI in particular, are a real game-changer. They speed up work, automate repetitive tasks, and completely change the way we think, create, and organize.
Can AI hallucinations put a dent in this picture?
It depends on what you want to get out of it.
If you just throw a bunch of makeshift prompts at ChatGPT and expect a masterpiece of literature to magically appear, well, brace yourself for a reality check. But if you use AI like any other tool, are aware of its limitations, and apply common sense, you will be fine.
Ok, let’s see what we learned today:
- 👉 Artificial intelligence hallucination refers to a scenario where an AI system generates an output that is not accurate or present in its original training data.
- 👉 AI models like GPT-3 or GPT-4 use machine learning algorithms to learn from data.
- 👉 Low-quality training data and unclear prompts can lead to AI hallucinations.
- 👉 AI hallucinations can result in blending fiction with reality, generating dream-like visuals, or causing problems in automated systems like self-driving cars.
- 👉 Hallucinations may raise concerns about the safety of AI systems.
- 👉 You can apply prompt engineering practices to minimize or prevent AI hallucinations.
And that’s it for today!
💭 Frequently Asked Questions About AI Hallucinations
What are AI hallucinations?
Artificial Intelligence (AI) hallucinations refer to an intriguing phenomenon wherein AI systems, particularly Deep Learning models, generate outputs that veer from the expected reality, often inventing non-existent or surprising details. Much like an artist filling an empty canvas, AI hallucinations illustrate the system’s creativity when faced with data gaps.
What causes AI hallucinations?
The primary reason for these AI hallucinations is the intrinsic limitations and biases in the training data. AI systems learn by processing large volumes of data and identifying patterns within that data. If the training data contains misleading or inaccurate information, or if it is unrepresentative of the wider context the AI may encounter in real-world applications, the AI might produce hallucinations.
What are examples of AI hallucinations?
AI hallucinations typically refer to situations where a machine learning model misinterprets or creates incorrect outputs based on the data it has been fed. For instance, an image recognition system might hallucinate a non-existent object in a picture, like perceiving a banana in an image of an empty room. In the realm of language models, an AI hallucination could be the creation of an entirely false piece of information, e.g. that the Eiffel Tower is in London.
Can AI hallucinations be prevented or reduced?
Yes, AI hallucinations can be reduced or even prevented through a combination of strategies. First, data used for training needs to be diverse, representative, and as bias-free as possible. Overfitting should also be avoided, which is when the model learns the training data so well that it performs poorly on unseen data. Finally, users can learn how to write effective AI prompts according to established prompt engineering best practices.
Why are AI hallucinations called “hallucinations”? Isn’t that term misleading?
The term “hallucination” in the context of artificial intelligence (AI) is indeed somewhat metaphorical, and it’s borrowed from the human condition where one perceives things that aren’t there. In AI, a “hallucination” refers to when an AI system generates or perceives information that doesn’t exist in the input data. This term is used to highlight the discrepancy between what the AI “sees” or generates, and the reality of the data it’s interpreting.
Are AI hallucinations a sign of a flaw in the AI system, or can they have some utility?
AI hallucinations, in most contexts, represent a flaw or error in the AI system, specifically related to how the system was trained or the bias in the data it was trained on. They can lead to misinterpretations or misinformation, which can be problematic in many practical applications of AI. However, some aspects of what we might term “AI hallucinations” can have utility in certain contexts. For example, in generative AI models that are designed to create new, original content, the ability to generate a unique output can be beneficial.
Can AI hallucinations lead to the spread of false information?
Yes, AI hallucinations can certainly contribute to the spread of false information. For instance, if an AI language model produces inaccurate or misleading statements, these could be taken as truth by users, especially given the increasing tendency for people to use AI as a source of information. This could be particularly harmful in fields like healthcare and finance.
Are certain types of AI or machine learning models more prone to hallucinations than others?
Some types of AI or machine learning models can be more prone to hallucinations than others. In particular, deep learning models, especially generative models, are often associated with hallucinations. This is because these models have a high level of complexity and can learn intricate patterns, which sometimes results in the generation of outputs not reflecting input data.